Gen AI in Finance Controllership – Recent Conversations reveal cautious approach to adoption
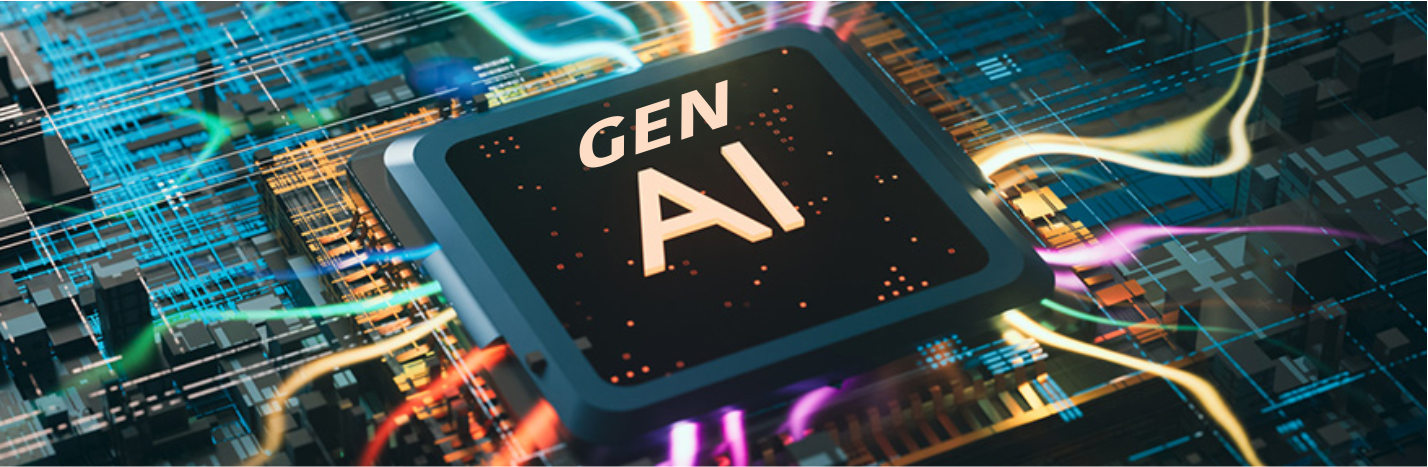
Gen AI is an exciting opportunity for companies to explore especially in the world of finance and accounts. There are plenty of use cases to adopt these models some of which I have attempted to list below:
- Finance close workstream – Gen AI models can be used to generate finance close task lists which can be especially useful for small to mid-market companies who are looking to streamline their finance close function or process. These task lists can be specific to industry and sector thereby significantly increasing the relevance of the check points which a finance controller will like to have in place.
- Flux management – Mid to large companies lay a lot of emphasis on flux management as a process especially given that a lot of analysis is carried out around unit metrics to gauge businesses’ performance. Gen AI can help in carrying out the initial data analysis and propose possible reasons for variance which users can review and add inputs. Over a period, the accuracy of these explanations will definitely improve thereby giving real time insights to stakeholders (CXOs) for carrying out quick and effective business decisions.
- Transaction anomalies – More often than not controllers and auditors require a keen eye on accounting transaction related anomalies. Whether those are non-recurring journal entries or unusual related party transactions the list of parameters for monitoring these anomalies are reasonably finite. AI models with these parameters can scan all the accounting records like general ledgers, sub-ledgers, control accounts etc and throw out all possible anomalies including possible explanations or exceptions for such transactions. This outcome can be reviewed by controllers thereby focusing on managing the exceptions a lot more thoroughly which otherwise can take a fair amount of time.
- Financial reporting – CFOs and controllers spend a lot of time in preparing financial reports and a major chunk of such time is spent in data gathering and collation. AI models, once trained, can carry out the grunt work surrounding data gathering and collation and can also simultaneously build up narratives allowing controllers to focus on the quality of information and insights.
The above use cases highlighted here are illustrative in nature based on the conversations we have had with customers. However as exciting as the use cases are there are clear risks which also need to be factored before deciding to adopt.
- Data Privacy and security is probably the biggest concern which finance teams have given that these AI models require a fair amount of data ingestion.
- Finance decisions require transparency and accountability and hence AI models have to be trained to justify the basis of its predictions etc. for stakeholders to be able to place reliance.
- Training AI models is not easy especially given that the data required for training has to be unbiased and fair as only then accurate outcomes can be generated by these models.
These are probably the top 3 concerns which customers face when they evaluate adoption of Gen AI models in their day-to-day operations withing the finance organisation. Hence a cautious approach and mindset is required to balance out the exciting possibilities vis-a-vis the presence of inherent risks when evaluating Gen AI models.